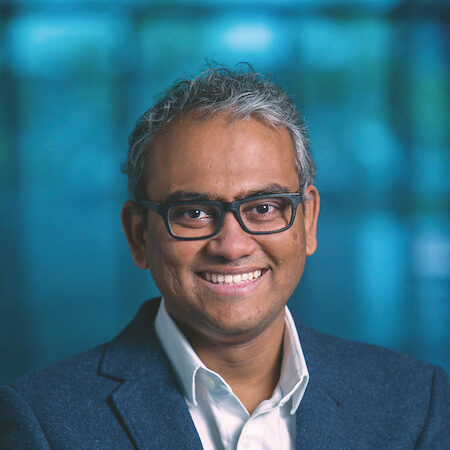
Each year, the Intel® Rising Star Faculty Award (RSA) program selects early-career academic researchers who lead groundbreaking technology research demonstrating the potential to disrupt the tech industry. The 15 award winners chosen for 2022 have presented impressive works in computer science, electrical engineering, computer engineering, material science, and chemical engineering.
The program proudly recognizes community members who are doing exceptional work in the field and hopes to facilitate long-term collaborative relationships with senior technical leaders at Intel. Award recipients are also chosen for their innovative teaching methods and for increasing the participation of women and underrepresented minorities in computer science and engineering.
The research conducted by the selected faculty provides novel solutions to challenges spanning various topics, including digital fabrication, quantum materials dynamics, cryptography, energy-efficient memory, microelectronics, computer architecture, deep learning, neuromorphic computing, and human-AI interaction.
Pratik Chaudhari’s goal is to understand learning in artificial and biological systems by identifying common themes in intracellular signaling networks of proteins, retinal circuits, and artificial neural networks. He proposes to investigate a characteristic structure called “sloppiness”. Sloppy systems are neither completely robust (insensitive to perturbations but difficult to adapt), nor perfectly tuned (efficient and precise but fragile). Instead, they exhibit a wide range of sensitivities. His work aims to show how sloppiness allows a system to strike a balance between doing well on one task and adapting to new ones. To accomplish this, Chaudhari proposes research organized into two main thrusts. First, examining how sloppiness can explain the performance of artificial neural networks, which could lead to a transformative understanding of the conceptual basis of deep learning. Second, studying models of the early visual system to understand how sloppiness emerges when circuits of neurons adapt to changes in the environment; this can inform the design of algorithms for neuromorphic hardware such as event-based cameras.