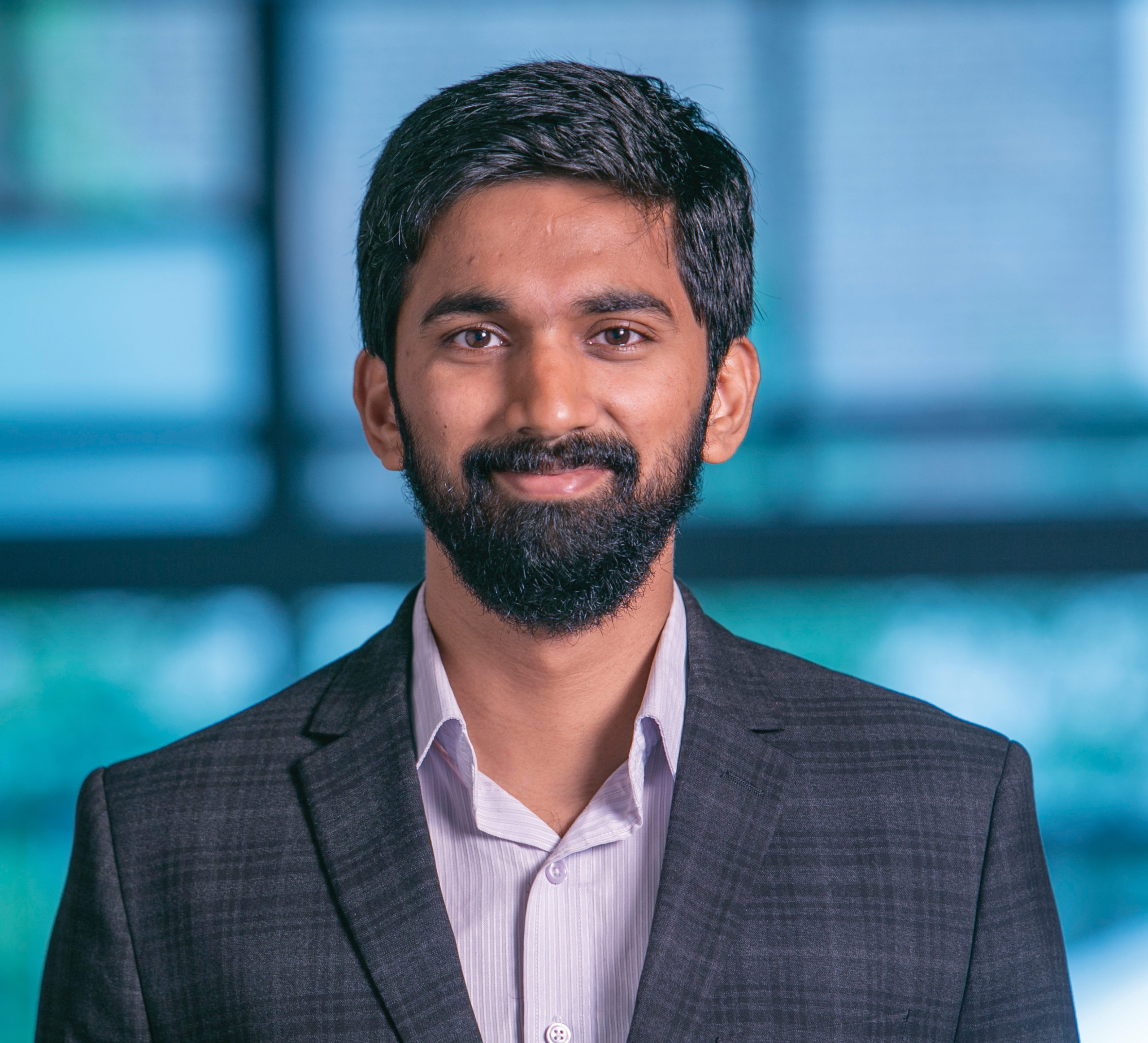
Text by Jillian Mallon
This article is one of a two-part series on the GRASP Lab’s involvement in the NSF’s new Safe Learning-Enabled Systems program. The article below covers the proposal that GRASP faculty member Dr. Dinesh Jayaraman contributed to in the synergy category. Click here to read the article that covers the proposal in the foundation category that was developed by GRASP faculty members Dr. Nikolai Matni and Dr. George Pappas.
Artificial intelligence-enabled platforms have become more widely available in a greater number of applications in the past year. Since the release of ChatGPT in November 2022, companies have launched their own AI platforms such as Google’s Bard and Adobe’s Firefly. Despite the growing accessibility, the reliability of these applications is still in question. Universities including the University of Pennsylvania have implemented guidelines for the safe use of predictive speech tools due to an increased risk of errors and plagiarism. This is just one reason the machine learning community has a need to develop ways to ensure the safety of AI platforms.
The National Science Foundation debuted its new Safe Learning-Enabled Systems (NSF SLES) program this year in search of ideas that would increase the safety of systems that are equipped with artificial intelligence. The competitive grant allocated its $10.9 million in funding in its inaugural year to only 11 projects across the nation in two different categories: foundation and synergy. Despite such a limited selection of winners, GRASP Laboratory-affiliated faculty members at Penn were awarded funding for one project in each category.
GRASP Professor Dr. Dinesh Jayaraman collaborated with three other co-principal investigators in Penn’s Computer and Information Science department to develop the proposal in the synergy category which was titled “Specification-guided Perception-enabled Conformal Safe Reinforcement Learning”, or SpecsRL.
The objective of the SpecsRL project is to create a reinforcement learning framework that uses high-level logical specifications, theoretical and empirical methods, and learning algorithms to increase the safety of a system enabled with machine learning capabilities. The group will test this framework by training a robot to complete complex kitchen tasks such as cooking pasta and setting a table.
The SpecsRL team, comprised of lead principal investigator Dr. Rajeev Alur as well Dr. Osbert Bastani, Dr. Dinesh Jayaraman and Dr. Eric Wong, was uniquely equipped to prepare this proposal due to their collaboration and research backgrounds. All four researchers have collaborated on the improvement of safety in learning-enabled systems as members of ASSET, Penn Engineering’s center for trustworthy AI. In fact, Dr. Alur is the founding director of the center.
The proposal also benefits from the combined knowledge of a diverse range of research methods. Each co-PI specializes in a different area of machine learning. “That’s partly the reason why the team was successful, because we came from slightly different viewpoints on this,” Dr. Jayaraman explained, “Rajeev has been working in the formal methods area for a very long time. Osbert is kind of straddling two disciplines – programming languages and machine learning, and he and I have already been collaborating on robotics problems. I am, of course, working in robotics and learning and vision, and Eric is interested in machine learning and robustness, so in some sense it was really the perfect team. It was really great that we had everybody at hand right here at Penn. I think that was one of the strengths of the proposal.”
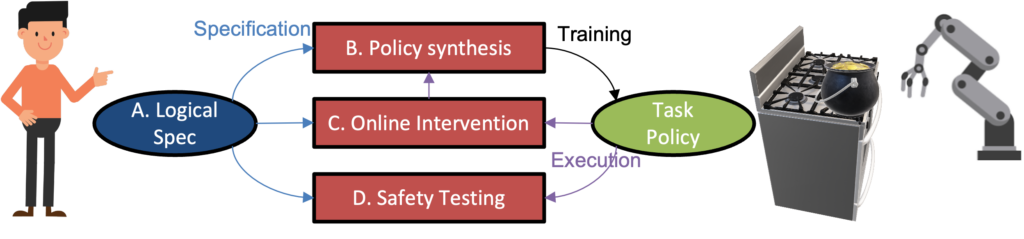
This proposal is made up of five main components. The first is to develop a logic-based specification language designed for programming the system with visual examples that explain the correct sequence of tasks and what must be completed and avoided in the process. The second component of the proposal is to develop methods to inform the system of potential uncertainties that cause safety risks.
“So we’ll say, ‘This is what it means to boil water’ or ‘This is what it means to not let water spill when you’re pouring it’,” described Dr. Jayaraman, “These kinds of visual examples will let the robot ground the words in the logical specification into something so that it can determine whether that thing is actually happening or not happening, and that will guide how it learns the task.”
Third, the team will equip the robot with robust decision-making abilities through the development of online monitoring techniques that will allow the robot to “check its work”. The fourth thrust of the proposal is to develop empirical stress testing techniques to uncover potential risks of failure. Fifth and finally, the fully developed approach will be evaluated both in simulation and by a robot in a mock kitchen environment.
“We pathologically construct these settings for you in such a way that your robot will fail if it was ever going to fail in that setting in the real world, and it’s much better for it to fail in the lab than in the real world. Part of the difficulty is even knowing where it would fail in the first place,” Dr. Jayaraman stated.
The SpecsRL team, eager to start their work, began the first stages of work on this project in October 2023. Eventually, the final testing of this project will take place in GRASP’s new mock kitchen space in Levine Hall that was constructed last year for use in cooking and kitchen-simulating experiments.
“I think that the kitchen is a large and important space for robots to eventually make a contribution. I think we’re probably going to get there in the next 10 years and hopefully our group will be involved as well as others at GRASP in pushing robots to get there,” said Dr. Jayaraman, “I’d be really delighted if some of our work, both over the course of this project as well as afterwards, led to my mother being able to use a robot in her home in 10 years and not having to worry about all the kitchen chores.”
While GRASP faculty members approached the NSF SLES call with different methods, they share the same excitement about the new possibilities that improved safety measures could bring to the field of artificial intelligence.
Dr. Jayaraman hopes that this research will make the use of artificial intelligence seem more trustworthy to the public. “We’re really hopeful that, just as the NSF had envisioned, this leads to a quantum leap. Hopefully, together with the other funded projects, including Nik’s project here at Penn, we can push systems along to the point where it’s actually more palatable to the general public, as well as to an AI researcher, that an AI system can be involved in safety-critical use cases,” he explained, “Many of the eventual applications through which we hope AI will take the burden off of humans do involve safety-critical components. There are potentially human lives at stake. That’s an important responsibility. As AI researchers we are certainly aware of this responsibility, but also the general public needs to have the trust to let a robot in their home, otherwise none of this is going to happen. Hopefully this will also help push forward the level of public trust.”