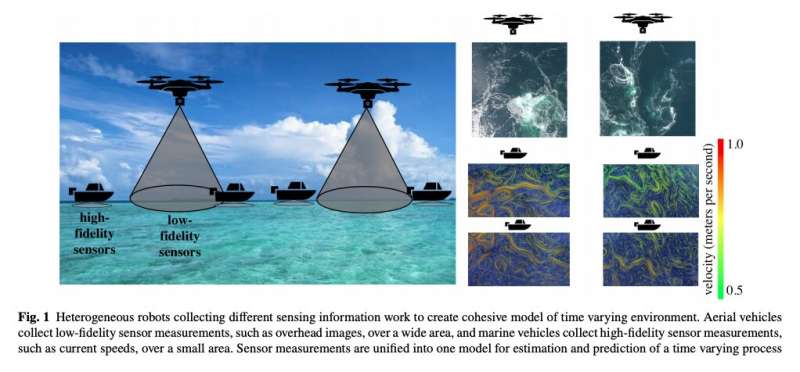
Published by TechExplore
Authored by Ingrid Fadelli
Photo provided by Salam and Hsieh
Teams of multiple robots could help to tackle a number of complex real-world problems, for instance, assisting human agents during search and rescue missions, monitoring the environment or assessing the damage caused by natural disasters. Over the past few years, multi-robot systems have proved to be particularly useful for solving problems that involve a distribution over space or time (i.e., allowing agents to cover large distances or monitor processes over time).
Researchers at the University of Pennsylvania’s GRASP Laboratory recently developed a framework that allows teams of robots to model environmental processes over time. This framework, presented in a paper pre-published on arXiv, could enable the use of multi-robot systems to predict the evolution of complex, dynamic, and nonlinear phenomena, such as forest fires, insect infestations, or dispersions of pollutants.
“We propose a coupled strategy, where robots of one type collect high-fidelity measurements at a slow time scale and robots of another type collect low-fidelity measurements at a fast time scale, for the purpose of fusing measurements together,” Tahiya Salam and M. Ani Hsieh wrote in their paper.
The framework developed by the researchers entails the use of two teams of robots with different patterns of movement and sensing capabilities (e.g., aerial, ground, and marine robots). As some environmental processes can be complex and multi-dimensional, these teams of robots can explore different dimensions and gather distinct measurements.
The researchers’ framework fuses the measurements gathered by two distinct teams of robots to create a model of complex, nonlinear spatiotemporal processes. This model can then be used to identify optimal sensing locations for the mobile robots and to predict how environmental processes will unfold or evolve over time.
“The framework presented allows for a decoupling of the temporal and spatial modes apparent in the data,” the researchers wrote in their paper. “This decoupling is then used within a task allocation framework for various types of robots. Instead of relying on the standard task-trait allocation approaches typically used by heterogeneous robotic frameworks, this approach leverages the unique strengths of the robots to jointly complete a task.”
Salam and Hsieh evaluated their framework in a series of mixed reality experiments. First, they assessed its ability to predict the evolution of an artificial plasma cloud. To do this, they created a simulated environment that replicates a plasma cloud in the vicinity of Earth. They then introduced four marine robots and two aerial vehicles into the simulated environment, which were supposed to gather different measurements and estimates associated with the evolution of the cloud.
The researchers used their framework to create a model that combined the measurements gathered by the simulated aerial and marine vehicles. They then compared this model’s predictions to those based on measurements collected by just one type of robots.
“Initially, the proposed heterogeneous approach performs comparably to using just the homogeneous marine vehicle data,” the researchers wrote in their paper. “The homogeneous data from the aerial vehicles is noisy and collected at much a lower spatial resolution than the true process. As the process becomes more complex, the inclusion of multiple types of data allows the proposed approach to outperform either of the other estimations.”
To assess its performance further, the researchers evaluated their framework’s ability to model the density of a different artificial plasma cloud projected inside a real water tank. In this experiment, they gathered measurements using three real micro-autonomous surface vehicles (mASVs), a simulated mASV, and two simulated aerial vehicles.
Overall, the tests carried out by Salam and Hsieh highlight the advantages of fusing measurements gathered by different types of robots to model complex environmental processes, rather than using measurements collected by a single type of robot. In the future, their framework could allow scientists to build unified maps or models of different environments, for instance, using aerial and marine robots to jointly map factors such as temperature or ocean currents.
Featured People
Deputy Director, GRASP Lab; Graduate Program Chair, ROBO; Associate Professor, MEAM
PhD, ESE '22 - VIAM